Research areas Heading link
Combined multi-physics and machine learning approach to forecast thermal runaway in LIBs
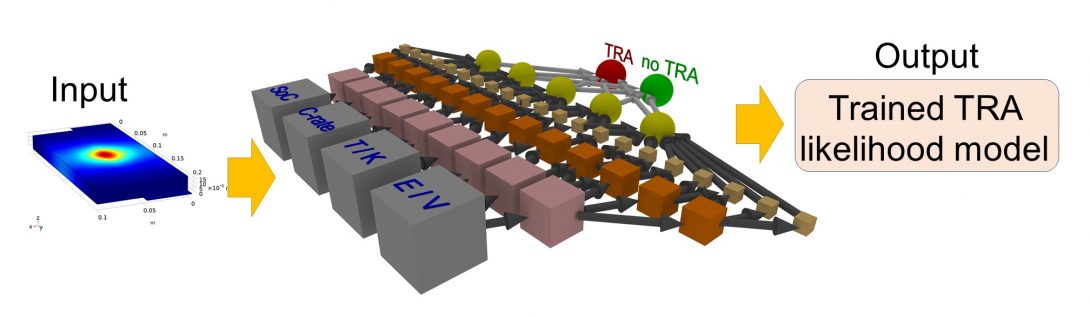
Over the last two decades, the tremendous effort of researches brought the Li-ion battery (LIB), based upon the intercalated graphite electrode, to its advanced level enabling their wide-spread application. Unfortunately, high demand led to many safety problems especially associated with thermal runaway. A very common reason of LIBs’ thermal runaway is the exothermic decomposition of solid electrolyte interface (SEI) leading to a battery’s rapid self-heating and in extreme scenarios to explosion. It is very challenging to suppress, control or sometimes even observe the thermal runaway due to the SEI formation/decomposition in realistic operation conditions. However, the evolution of thermal and electric parameters during LIBs operation may indicate the occurrence of thermal runaway, which will allow its early forecasting and corresponding suppression.
In order to predict the thermal runaway in LIBs, we use machine learning (ML) techniques, in particular, convolutional neural network (CNN) and deep neural network (DNN) to identify temperature pattern change leading to the thermal runaway. The ML methods are trained based upon the images obtained from the multi-physics calculations using Comsol software package. The pouch type LIB consisting of five distinct layers, i.e., two current collectors, negative and positive electrodes and electrolyte, is considered. The electrochemical model is based on a 1D continuum description of reaction and transport along electrodes, electrolyte and current collectors, plus an additional dimension in the electrode particle (P2D). The SEI formation/decomposition is modeled using the parasitic current approach. We investigate three regimes of thermal runaway occurrence, i.e., a single heat source, two heat sources and multiple sources. Corresponding, different CNN and DNN architectures were built and trained based upon the images from multi-physics calculations. The prediction of the trained network has been tested using simulated and literature available data.
The investigation of Li dendrites formation and growth in Li-metal batteries employing PFM and DFT calculations
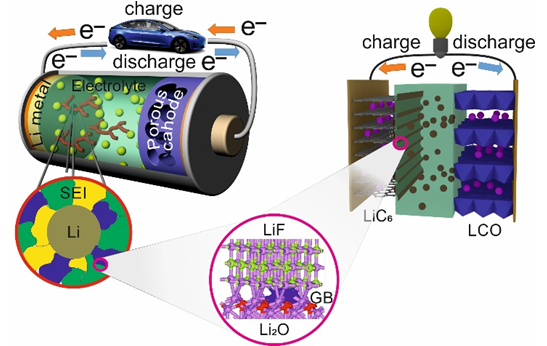
Using a phase-field modeling (PFM) study of lithium (Li) electrodeposition process in Li‒metal battery (LMB) to explain its physics and morphology. Three regimes are investigated: Li filaments evolution, Li bush-like structure evolution and the transition between Li filaments and bush-like morphologies. The model takes into consideration the effect of solid electrolyte interface (SEI) on Li electrodeposits evolution. Also, an important new element of the model is the capability to represent the directional diffusion of Li by implementing diffusion tensor of Li-ions in the electrolyte, thus, allowing to simulate Li filaments root growth. In addition, an elastic deformation energy of the Li solid phase is included in the free energy functional of the PFM, which allows for monitoring the stress field and its influence on Li filaments/bush-like structure evolution. In particular, a significant stress is observed at the root of the Li electrodeposits, which can support the development of the experimental strategies to suppress their formation by lowering the stress field. Thus, the present study, in addition to improving the fidelity of the PFM of Li electrodeposition, identifies critical regimes of Li filaments growth and splitting, allowing for a more profound understanding of their influence on battery performance.
The investigation of Zn dendrites formation and growth in Zn batteries employing PFM and DFT calculations
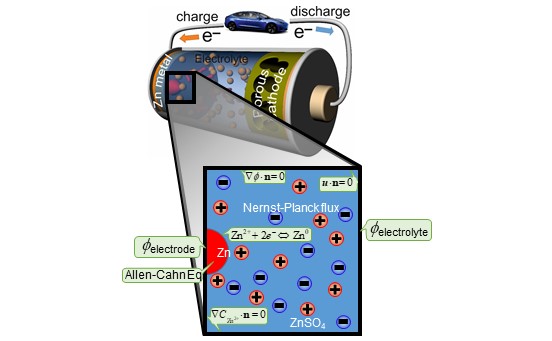
Zinc (Zn) aqueous rechargeable batteries (ZB) have showed a tremendous success in various applications, however, analogous to their Li-ion battery counterparts, they suffer from the dendrite formation and eventual decrease in capacity. In our current work, we present the results from phase-field modeling (PFM) of Zn electrodeposition at different current densities. Different Zn morphologies, such as boulders, whiskers and dendritic shape are modeled. The computational model predicts two-dimensional distribution of Zn electrodeposits, Zn2+ ions concentration, electrostatic potential, stress and equivalent plastic strain. It was found that the stress has a major influence on the electrodeposition, and vice versa the Zn electrodeposits growth is found to affect the stress distribution significantly. The plastic yielding occurs preferentially at the node of the boulders and through the filaments and dendritic structures. The results also provide a basis for development and design of novel strategies against unwanted Zn dendrites formation.
Modeling Elasto-Plastic Deformation in Lithium-ion Batteries: Si electrode
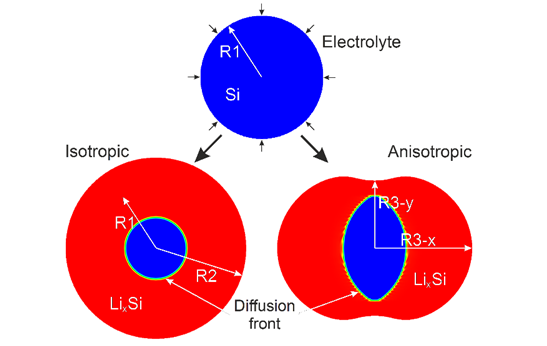
Modeling Elasto-Plastic Deformation in Lithium-ion Batteries: Si electrode
The understanding of elasto-plastic deformation is crucial for performance and durability of Lithium (Li) ion batteries. In our group, we are developing a generic framework to describe kinetics of alternative electrodes (e.g. Silicon) lithiation and its effect on structural changes, stress evolution and mechanical properties. Two models have been developed, where the first approach is a coupled finite element elasto-plastic model using ANSYS finite element package, and the second is a phase-field model based upon MOOSE framework. The models include the description of the lithiation induced phase transformation, morphological evolution and stress generation in Si nanowires.
Bi electrode
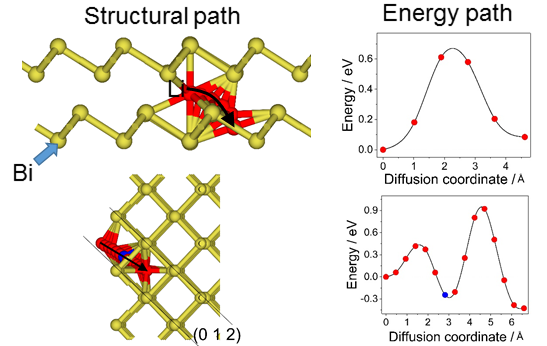
DFT calculations of (de)alloying in Lithium-ion Batteries: Bi electrode
Materials storing energy via alloying reaction are promising anode candidate in rechargeable lithium-ion batteries (LIBs) due to their much higher energy density than current graphite anode. Among these materials, bismuth (Bi) provides a high theoretical volumetric capacity of 3800 mAh·cm−3 when it alloys with Li+ forming LixBi composites. Using the DFT method, we studied the favorable pathways for Li diffusion into the bulk of Bi as well as the intercalation path from the most stable surface into the bulk of Bi.
Phase transition in LCO cathode
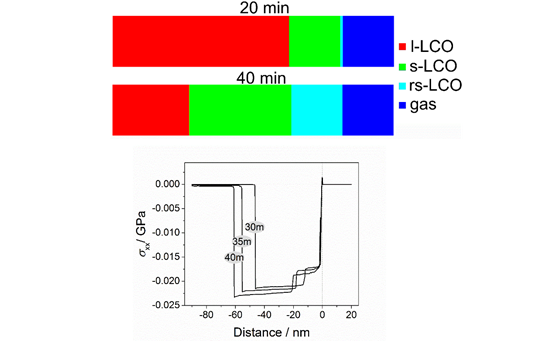
Despite the tremendous success of Li-ion battery based upon liquid electrolytes and oxide positive electrodes, their widespread application is limited due to the safety concerns originated from the oxygen release. Consequently, the oxygen release causes phase transformation, which also leads to the mechanical failure of a battery. We have developed a detailed multiphase-field model (PFM) to predict chemo-mechanical properties of oxide based battery electrodes. The PFM considers the chemical composition change, the associated phase transformation and the stress generation in the bulk as well as at the surface of the electrode. This model is applied to capture the development and evaluation of phase transformation mechanism, which occurs at elevated temperatures in the partially delithiated Li0.45CoO2 (LCO) material. Our results indicate that the major oxygen concentration change occurs in the narrow region between the phases, and the compressive stress is generated inside the bulk LCO, whereas tensile stress is observed within the LCO-gas phase interface. In addition, an important contribution of this work is the derivation of a new set of thermodynamic and kinetic data of the oxygen release. Thus, our findings provide new qualitative and quantitative understandings of the LCO phase transformations and the kinetics of oxygen release.
Defective structure investigation in NMC cathode
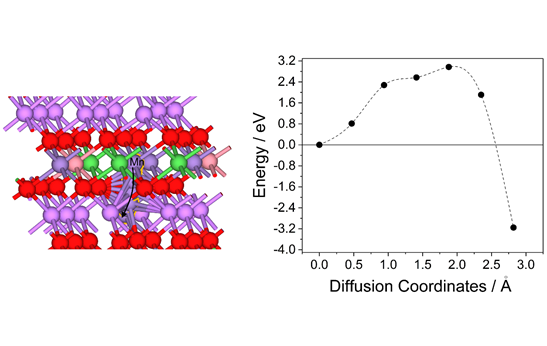
Despite the high energy density, challenges such as rapid capacity degradation and voltage decay have limited the widespread application of Li-rich layered cathode (LLC) materials. Thus, it is critical to fully understand the degradation mechanisms that contribute to the lower structural stability of Li-rich cathodes compared to the conventional layered oxide cathodes. We use density functional theory (DFT) calculations to investigate atomic arrangement of metals in the NMC cathode to reveal degradation mechanism of these materials.
Modeling of the Electrochemical Performance of Lithium Batteries
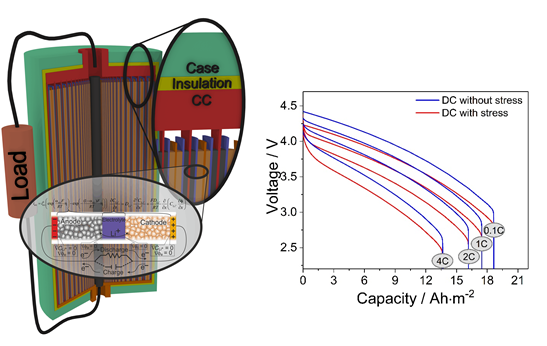
We use COMSOL software for the assessment of the electrochemical and degradation phenomena in the continuum modeling approach
Convolutional Neural Network (CNN): Identification of atomic column heights and defects in experimentally obtained HRTEM images
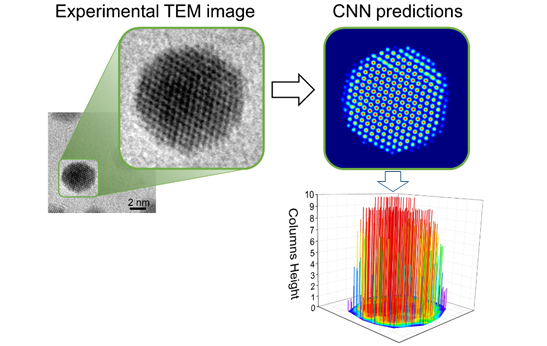
Transmission electron microscopy (TEM) provides the highest spatial resolution to an image of the nanoparticle’s atomic structure, it is time consuming and cumbersome to estimate the atomic column heights from the two-dimensional projected TEM images. With continued progress of in-situ or operando TEM techniques in discovery of nanoscale science, it is paramount to develop artificial intelligence approaches that can be integrated with real-time TEM imaging. In this work, we present a modeling framework based upon deep learning approach (i.e., convolutional neural network-CNN) for the detection of the atomic column heights in the experimental high-resolution transmission electron microscopy (HRTEM) images.
Recurrent Neural Network (RNN): Battery management system and electric vehicle
We use machine learning techniques (e.g., recurrent neural network) to study battery performance in application to electric vehicles (EV). In particular, the train our model to predict the state-of-charge (SOC) as used in the battery management systems (BMSs). Matlab/Simulink coupled to Comsol multi-physics software is used to generate the training data set. Consequently different driving cycles are utilized to test the model and predict SOC in complex conditions.
Data driven estimation of electric vehicle battery state-of-charge
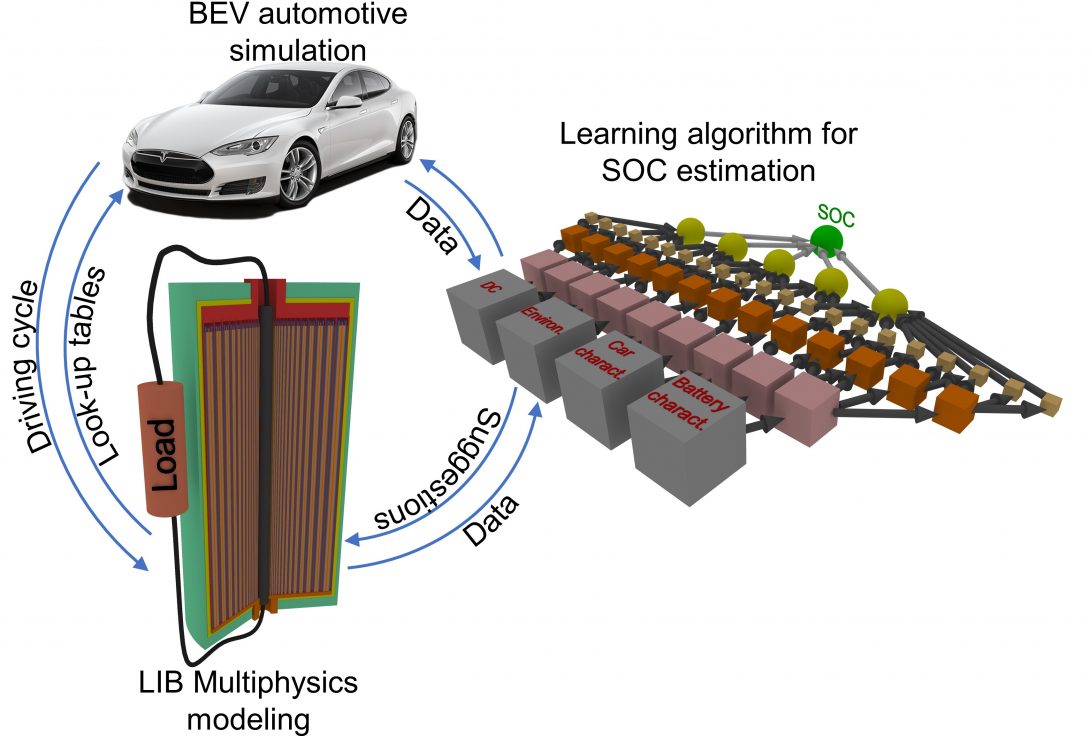
State-of-charge (SOC) estimation in a lithium-ion battery (LIB) is a crucial task of the battery management system (BMS) in battery electric vehicle (BEV) applications. In this work, we propose a modeling framework for SOC estimation using different machine learning (ML) methods, i.e. support vector regressor (SVR), artificial neural network (ANN), and long-short term memory (LSTM) network. The necessary training data have been developed using Matlab/Simulink automotive simulations of BEV, integrated with an electrochemical Comsol Multiphysics model of LIBs. The developed multi-physics model of BEV and LIBs operation allows to investigate the effect of driving conditions on the electrochemical and degradation (i.e., the solid electrolyte interphase – SEI – formation and decomposition) processes occurring inside batteries of different chemistries adopted in the Tesla S and Nissan Leaf BEVs. Our study remarks also the importance of taking into account the different components of BEV in the development of informative datasets, which are required for the implementation of learning algorithms for SOC evaluation. Thus, the proposed work establishes a basis for the generation of realistic training data based on simulations of BEV and LIBs dynamic response, which allows a more precise SOC estimation based on data driven approaches.
Research methods Heading link
Phase-field modeling and software development
MOOSE (Multiphysics Object-Oriented Simulation Environment) is a finite-element, multiphysics framework, which provides a high-level interface to some of the most sophisticated nonlinear solver technology. The MOOSE framework was developed to simplify the creation of fully coupled, nonlinear, multiphysics applications, where each application is made up of physics “modules” that describe the PDEs to be solved, material properties, boundary and initial conditions, postprocessed quantities, etc. In our work, we use MOOSE for the phase-field based numerical simulations.
Finite element model development in Ansys
Finite element model development in Ansys.
The species concentration and stress–strain fields are solved with an implicit, coupled temperature–displacement procedure in ANSYS®. Since the governing equations of the slow chemical stress are mathematically analogous to those of thermal stress, they are solved by treating the chemical source as a thermal source. The species ion diffusion is considered analogous to heat diffusion, and the expansion coefficient is equivalently treated as the thermal expansion coefficient. An 8-node quadratic coupled field element (PLANE 223) is used to accommodate both the structural and thermal degrees of freedom. The element has eight nodes with four degrees of freedom per node. The finite deformation effects due to diffusion, geometric nonlinearity and material nonlinearity are considered by using a large deformation formulation and bilinear isotropic hardening material model respectively.
Finite element model development in Comsol Multiphysics
Finite element model development in Comsol Multiphysics.
Different modules of Comsol are used to solve physico-chemical problems of interest. In particular, Li-ion battery, battery management system, etc.
Matlab/Simulink model
Matlab/Simulink model is developed to simulated electric vehicle performance.
Density functional theory (DFT) calculations
Density functional theory (DFT) calculations.
For our DFT calculations we use the VASP (Vienna Ab initio Simulation Package) code and the Atomistic Tool Kit (ATK) ofr creation of structures and NEBs.
Machine learning
We use open source codes and libraries such as Keras, Theano, TensorFlow, etc.